Analytics is the process of applying math to data to get insights into specific questions. Data Analytics has become a diverse and all-encompassing tool that deals with the extraction of usable information from data, with the goal of improving systems. In any discipline there is a need for information regarding trends and metrics that increase understanding and efficiency. For this article we are going to restrict our view to that of data analysis for businesses.
Critical data is usually stored scattered, lost in a sea of folders and files that would render it useless. Most industries collect data in large amounts with wildly varying forms and methods of storage. The greatest barrier for analytics is unorganized data. It is imperative for companies to store their data effectively for analysis to be utilized. This information can be tracking a myriad of quantities such as: customers visits, sales, KPIs (key performance indicators), machine diagnostics, or even reviews. Applying analysis to this data can be endlessly useful. It is important for companies to have easy access to data for analysis or quickly fall behind in this digital age.
Data analytics falls under four types which include, descriptive, diagnostic, predictive, and prescriptive analytics. Each type of analysis is used for specific purposes. Companies need to know the how, what, where, and why to manage their businesses and allow for better decision making. Analysis done correctly provides insights that give a clear competitive advantage.
The Different Types of Analytics
Descriptive Analytics is answering what happened. Descriptive analytics in its most common form is running reports. Compiling raw data to answer questions of how much/many or even when past events took place falls under descriptive analytics. Reporting questions look like:
- When was our busiest month?
- Who sold the most last week?
- How many items did we produce?
Knowing what has happened provides valuable insights and is a good measure of a company’s current health. However, using descriptive analytics to predict and explain events subtly shifts a question to intuition that can be better answered with analysis. Descriptive analytics is a necessary first step in analysis, but it should not be your last.
- Diagnostic Analytics is answering why something happened. This is accomplished by finding connections between different types of data. Diagnostics is an involved process requiring large quantities of data. Analytics alone tells if two events are related but knowing if one caused the other often requires more information.
- Example: Imagine tracking ice cream sales and pool visits. The two are related, when visits are up, so are sales. To say pool visits cause ice cream sales, or vice versa, would be silly. A better explanation would be sales and visits are caused by daily weather/temperature. Causation for both sales and visits were something outside the data available. In this example knowing the relationship told us nothing of their cause.
Diagnostic analytics is a very useful tool that should not be discounted. Establishing the causal relationship requires large amounts of data along with using more advanced statistical methods. Once the causal relation is known the trends of one data set completely informs on the trends of the other and thus has immense predictive power. Diagnostics is a needed first step in doing advanced analytics and really shines when used in combination with predictive analytics.
- Predictive Analytics is answering what might happen. This analysis is accomplished by using data from the past to make predictions about the future. Some questions that are answered by this analysis are:
- What will sales look like next month?
- When will our busiest day be next week?
- When will this machine break down?
Predictive analytics is a wildly useful tool to have in your arsenal, but only when viewed with the appropriate level of skepticism. Knowing when you can trust predictive analytics is a very useful skill to have. Undeniably this analysis cannot go understated, even when predictive analytics lacks reliability, like when chaos is present. There are two types of chaotic systems that can cause problems:
- An instance of large unpredictability with a return to a new normal afterwards (think forecasting models at the start of the pandemic). This system just needs a model adjustment before analysis becomes useful again.
- A system whose values we are trying to predict are largely determined by pure randomness (think the stock market). This system resists all attempts to be modeled.
In lieu of its limitations when predictive analytics works it is thrilling. Predictive analytics is an invaluable resource that should absolutely be in any decision maker’s tool belt.
- Prescriptive Analytics is answering what should be done. This is by far the most involved and complicated analytics mentioned so far. Prescriptive analytics sits at the forefront of data science. Utilizing all forms of analytics mentioned above, prescriptive analytics takes the next step and asks what my best course of action is.
- Example: If you have ever played games like go, checkers, chess, ect… on the internet or in an app you will find this analysis familiar. A form of prescriptive analytics is when a move is recommended. What’s being done is (1) The configuration of pieces on the board is looked at and the possible/probable moves for your opponent are calculated (2) Your moves are looked at and then the favorability is calculated for each possible move. Based on all this information the best move is suggested.
Not surprising, machine learning is the core method of solving problems. With machine learning sitting as a gatekeeper, one would expect this to be an impenetrable form of analytics. This is not so anymore because there has been considerable work to lower the barrier to entry. Maximizing the effectiveness of the analysis still requires considerable knowledge. Prescriptive analytics is a very promising tool, but the high price point is something to consider.
Why is Analytics Necessary?
The ultimate goal for any business is profit and reducing cost. Data Analytics is a cost-effective way to maximize profits and efficiency. Ranging from basic reports to complex machine learning algorithms, companies big and small are adopting analytics. As a guardian, analytics stands against biased thinking and misleading intuition. Analytics stands to elevate the quality of information available to you; information being the cornerstone of decision making.
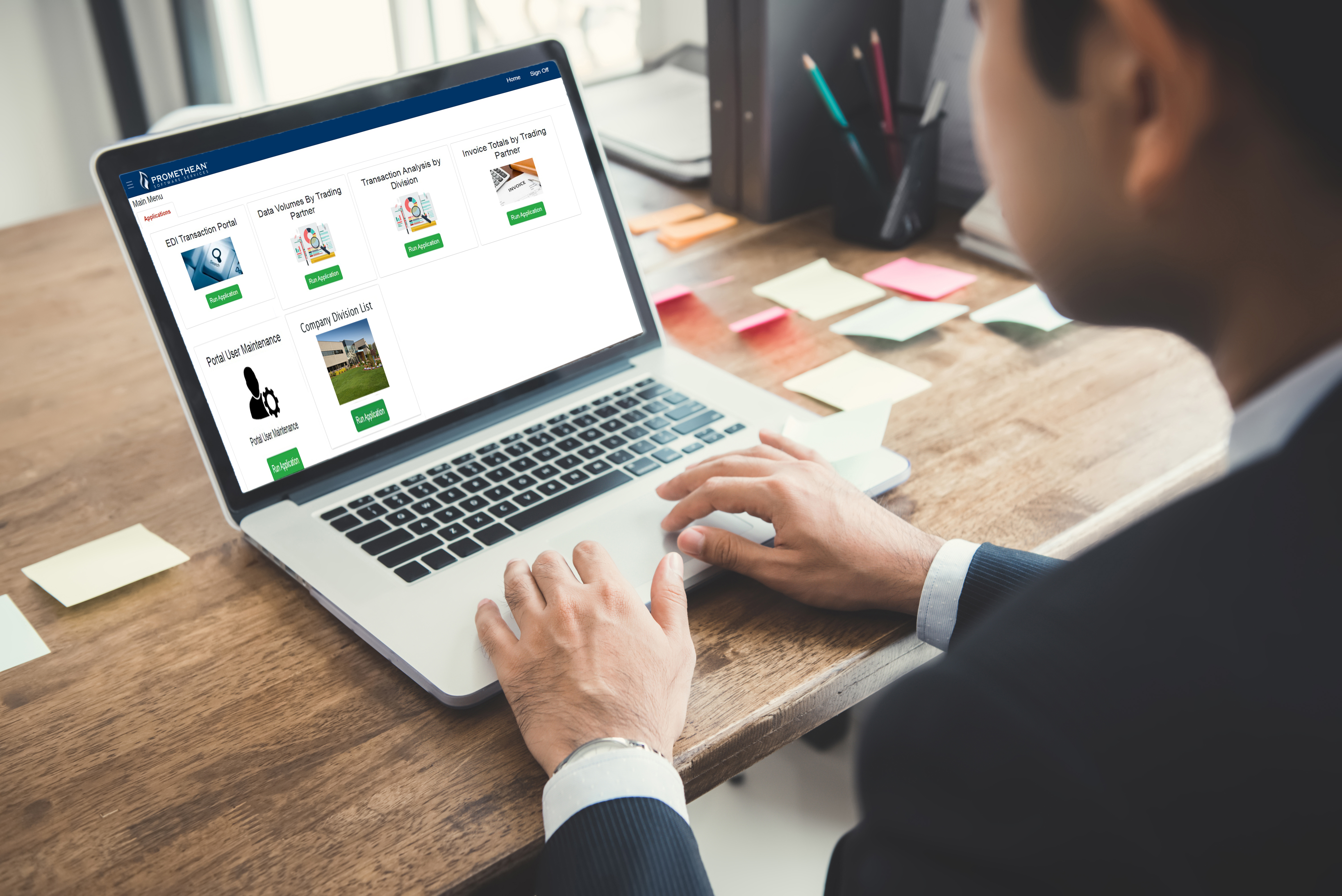
Many facets of industries benefit from data analytics. One of the clearest cut cases of its’ benefit is in dealing with the logical fallacies that plague any decision maker’s thinking. Some common logical fallacies that would stand as traps are:
- confirmation bias
- survivorship bias
- observational selections
- probability of small numbers
- Cherry Picking
- and the list goes on
The best safeguard we have against logical fallacies is understanding them. Letting the non-biased view of analysis answer questions unabated is the fulfillment of this understanding. Carl Sagan notes, on pg 34 of his book The Demon-Haunted World, Science is forever whispering in our ears, ‘Remember, you’re very new at this. You might be mistaken. You’ve been wrong before.’ Applied correctly, numbers cannot lie. Intuition alone is not sufficient, but intuition aided by the clear sight that analysis provides is formidable.
Benefiting from Analytics
The results of well-done analytics cannot be understated. It is vital to enhance the quality of decision making if you are to ensure the longevity of your business. Performing analysis is as much a mindset as it is a mathematical discipline. Retraining your mind to think of questions in terms of data to be gathered is a crucial first step. It is difficult to see the relationship between data and the questions it can answer. As far as I know there is no formulaic relation. This is a skill that will take practice and no small amount of curiosity. In the pursuit of maximizing the benefits of analysis a vital ally will be fostering curiosity in abundance.
You can always dig more, there will always be more to learn. To that point, it is easy to get carried away here. Running a business means being acutely aware that not all analysis leads to profitability. To that end, plan ahead. Have a goal for the questions you want to answer and establish a plan of how you are to accomplish it. Setting a deadline will help know when it is time to ask the next question. There will always be more questions to ask, and always room to optimize. Cultivate curiosity, and let that curiosity be data driven.
How to Start Doing Analysis?
The best thing to do is start. Use what you’ve got, where you’re at. If you don’t have data, try thinking of questions and start collecting. Excel is an excellent tool to start storing data in a readable fashion. When you start data collection make sure to store everything in the same format, you will thank yourself later. There is always the option of utilizing service providers to handle your data for you. Professional data storage companies take the hassle out of designing and storing data with the added benefit that most have reporting shown through interactive dashboards.
If you have data that is just sitting, try running reports. It may be the case that your data is not centralized or is not in a usable format. To that end, it would behoove you to get it organized so that you can utilize the full effectiveness that data offers. Otherwise, you are just wasting money on storing it. Again, Excel offers a lot of utility here with built in math functions and tools for making customized graphs and figures. There is other software available for this style of reporting and each has its merits. The best you can do is pick one and dedicate time to becoming efficient with that software. If this sounds like a hassle and you want to see the results sooner, professional data storage companies often have live dashboards that allow you to view your diagnostic data with ease. If you are already running reports, you can take the next step and start making predictions. This will allow you to start planning ahead and move you away from being reactionary.
Analytics is a collaborative journey; you shouldn’t venture alone. If you want a hands-off approach, there are companies out there that specialize in meeting you where you’re at. If you take a hands-on approach to the analysis, there are a myriad of blogs, YouTube channels, and communities centered around doing data analysis. Finding them and getting involved will do nothing but help. The true barrier to entry is the limit of your curiosity.
Contact us to discover how Promethean’s services can help your company generate and use data analytics today.