What is Prescriptive Analytics?
Prescriptive analytics is the next step in the analytics journey. It’s the stage where we consider what should be done. This type of analytics uses mathematical tools to suggest decision options based on earlier analytics. We build these models using descriptive, diagnostic, and predictive analytics. The key to creating effective prescriptive analytic models is identifying a specific question and the actionable variables that influence it. From there, a model can be constructed, and the variables determined inform the best course of action.
For instance, consider the question, “How can we maximize customer retention rates?” To answer this, you must first determine your retention rates using Descriptive analytic methods. Then, using diagnostic analytics methods, you can identify the variables that influence the retention rate, such as age, quarter (time of year), order quantity and price, or the time it takes to order a product, etc… From this list of independent variables, you can identify the actionable variables. These are the ones the company can directly influence, such as the age of the clients you advertise to, not the age of the clients you attract. Finally, predictive analytic methods are used to build a model that predicts future values of retention rates based on variables found using descriptive analytics. Prescriptive analytics then constructs another model that recommends optimized values for the actionable variables; in our example, this means finding the values that maximize retention rates. If the time to order a product is crucial for customer retention, then prescriptive analytics would help find the optimum time to complete an order to maximize retention. This could then guide where costs should be allocated to meet these goals. This leads to another example of prescriptive analysis: based on expected payouts and risk, how do you distribute money to optimize spending? The possibilities are endless, and there is always mathematical rigor to enhance decision-making.
Techniques and Methods Used in Prescriptive Analytics
The world of prescriptive analytics is vast and versatile, with many techniques and methods at your disposal. The choice of tools depends on the question you’re trying to answer. Here’s a glimpse into the diverse toolbox of prescriptive analytics:
· Game Theory is a branch of mathematics that deals with strategy analysis. Specifically, it concerns the Strategy of competitive situations in which groups’ actions affect each other. Game Theory is used in various ways and is highly nuanced; its applications range from business and economics to sociology. In general, game theory can be used to suggest your best course of action when competing with groups that are behaving rationally.
· Statistical/Probabilistic modeling can be seen as an extension of the techniques used in predictive analytics. Modeling refers to building a mathematical framework of the processes you are studying. For example, a model studying future sales might consist of a moving average over previous quarters used to predict the next, which is predictive analytics. Statistics and Probability find their way into modeling by accounting for inherent uncertainties in the values informing the model. This means accounting for randomness in the reported values and using that to inform future model values better. This relates to prescriptive analytics by simulating different values for your actionable variables within the statistical model to determine optimum outcomes.
· Machine Learning refers to building a statistical model that learns from data and generalizes to data that coheres to the same patterns. The distinction between machine learning and statistical/probabilistic modeling is based on a difference in methodology and scale. Statistical/probabilistic modeling is done piecewise and is used for problems with a smaller variable count. These models are built out in steps and are tested at each step. Machine learning is done all at once, with testing typically done at the end of the learning process, and it is used with a large variable count. When to use one vs the other depends on the scale of the project and the resources you wish to invest.
· Mathematical algorithms are used for narrowly defined questions about optimizations, min/maximizations, stochastic/random processes, etc. These methods are used when you have a rigorous understanding of the underlying mechanisms that you are trying to work with. This can range from designing optimal layouts of factory floors for increased productivity to selecting routes to minimize delivery times.
This list is not comprehensive but is meant to provide a starting point for learning about different methodologies. The distinctions between the categories can sometimes be unclear due to significant technique overlap. The differences usually come down to how the methods are implemented or their scale. This confusion arises from the cohesive nature of mathematics; it is a unified whole, and distinctions can become blurry when one way of categorizing and naming overlaps with another. However, this complexity is advantageous. It means that the math we learn has broad applicability when solving problems. It also means that there is typically no single correct way to solve problems, which is to our benefit. Having multiple approaches to problem-solving means that we can compare solutions across methodologies, which allows us to establish more confidence in our findings. When starting, due to the inherent complexity of the topic, it is preferable to seek the guidance of an expert after identifying your business questions.
Benefits of Prescriptive Analysis
The benefits of prescriptive analytics are significant. While other forms of analytics may leave you wondering what to do with the information they provide, prescriptive analytics addresses that very question. It involves making decisions with the confidence that comes from rigorous mathematical analysis, knowing that you’ve made the best decision with the available information. This doesn’t guarantee 100% accuracy or success, as it’s based on potentially incomplete or incorrect data. However, to repeat it does mean that you’ve made the best possible decision with the information at hand, giving yourself the highest chance of success.
Difficulties with Prescriptive Analysis
Prescriptive analytics sacrifices ambiguity for complexity. The complex analysis models and methods take work to understand and just as much to set up, which means a long investment before payoffs become apparent. Due to the complex nature of the analysis, an expert is essential to obtain results with any degree of confidence, which can be expensive. Collecting and storing data is also costly; the actual storage cost is not negligible with the increasing complexity of the solved problems. Determining the questions to ask to get started can also be challenging. This is only a problem at the start; curiosity begets curiosity, and once you begin asking questions, identifying new areas to investigate becomes second nature. Despite the associated costs and time, as your business grows in complexity, it becomes necessary to address decision-making with the clarity analysis provides.
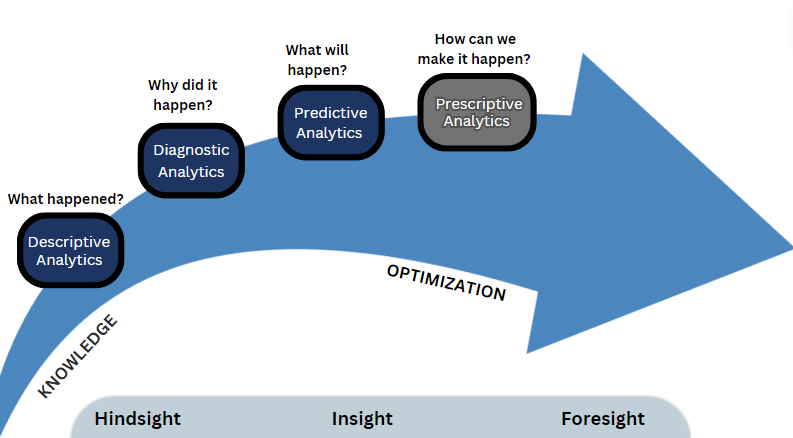
What Prescriptive Analysis Looks Like in Practice
Because prescriptive analytics is built per question, distilling it into a formulaic set of steps is difficult. So, instead of discussing how to solve an example problem, let’s consider when prescriptive analytics can be utilized and how to find actionable questions from the data. Whether you have a question in mind or not, familiarizing yourself with the data available is critical.
When looking at your data, consider the ranges present per variable. If a variable only has a single value or a small range, it is impossible to extrapolate to find optimal values in that variable. For example, consider the problem of finding the optimum price to sell a product with information about the price and previously associated sale quantities. Based on this data, the product must have been sold at different prices to find an optimum price. Another way to conceptualize this is to remember you cannot make a line from a single point; you need at least two points to create a line. There are other ways to calculate the optimal price, but it requires information outside of the data provided. Considering what your data can tell you significantly informs the questions you can ask.
Another factor to consider is the constraints on the variables. Constraints are anything that restricts a variable’s value. For example, you cannot sell something for a negative value, or there might be a minimum value that a product can be sold for. Another common constraint is how one variable might depend on other variables. Having equations that describe the dependencies can simplify the problem, but having too many can make the problem unsolvable. Knowing the constraints present for your data informs whether questions about that variable can be answered.
Additionally, randomness in the variables is essential to consider. A variable more determined by chance changes the framing of questions asked. Randomness changes questions to be more probabilistic. This means looking for ranges and distributions instead of values of variables.
This has been more general advice on using data to guide your questions. Prescriptive analytics deals with what we should do, given the data available. This means primarily thinking about optimizations and strategies. For example, let’s say you performed some analysis on your client base to determine the target demographic for a product. For the sake of the example, let’s also say that the target demographic fits nicely into a few categories (age ranges, incomes, location, etc.). Questions you might consider include:
· How do we split the advertising budget among the demographic categories to maximize clients?
· If a competitor is advertising to the same demographic category, how should you adjust your advertising strategy to maximize the return?
Prescriptive analytics focuses on utilizing information provided by other analytic methods. So, even asking, “How can we use this information?” is a prescriptive analytics question. However, having more precise and actionable questions yields a more straightforward analysis with unambiguous answers.
Conclusion
Prescriptive analytics is a crucial and intricate tool for decision-making. It enables companies to maximize the usefulness of their data and achieve the best possible outcomes. Despite the costs and complexity involved, exploring the potential of prescriptive analytics is always worthwhile.
Not sure where to begin with Prescriptive Analytics? Contact us to learn how utilizing your data can help you predict business trends, improve business decisions and gain a competitive advantage.